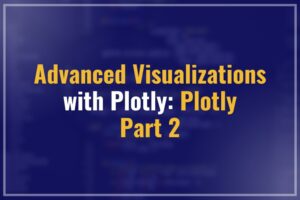
Greetings, everyone! I’m Althaf from 1stepGrow. Today, we’re venturing into advanced Python territory: regular expressions. This topic is a gem in Python’s arsenal. If you haven’t explored our earlier blogs, take a peek. But now, the wait is over. Let’s plunge into the much-awaited realm of regular expressions.xt
In this section, we will explore the fundamental building blocks of regular expressions, setting the stage for a deeper understanding of this powerful tool.
Regular expressions, often abbreviated as regex, are versatile patterns used for searching, matching, and manipulating text. They provide a concise and flexible way to describe complex text patterns. By leveraging a set of characters and symbols, regex enables you to pinpoint and manipulate data within strings efficiently.
By using regular expressions, you can quickly and precisely pinpoint various elements. Consider the following scenarios:
In essence, these data types possess distinctive patterns. Regular expressions act as search algorithms, comparing input to predefined patterns to identify correct formats. This proves indispensable for tasks like confirming the accuracy of user-provided email addresses, password strength, or valid phone numbers before submission.
The re module, short for regular expression module, equips Python with a powerful API for handling regular expressions. With this module at your fingertips, you gain the tools to work with intricate text patterns effortlessly.
At its core, a regular expression (regex) is a string designed to extract specific information from given data. This module serves as your gateway to the world of regex, enabling you to locate, match, and manipulate text patterns.
Now let us take a look at a simple example:
In this example, the regular expression r’c\w\w’ targets words that start with ‘c’ followed by two alphanumeric characters. The re.findall() function extracts all matching instances from the provided text. The output showcases the matches found: [‘cat’, ‘car’].
A regular expression is always written with r at the beginning which means this is a raw string format
Sequence characters in regular expressions enable you to precisely target specific types of characters or ranges within text. By understanding and leveraging these sequence characters, you gain the ability to manipulate and extract data with enhanced accuracy. Let’s dive into the world of sequence characters and learn how they can be harnessed to your advantage.
Sequence characters in regular expressions allow you to match and manipulate specific types of characters within text. One of their fundamental uses is to match single characters, providing a powerful tool for text processing.
Leveraging these sequence characters grants you the ability to effectively locate and manipulate individual characters within a text, streamlining your data processing tasks.
Let us take a look at an example to better understand this:
In this example, different sequence characters (\d, \w, \s, and .) are used to match and extract specific types of characters from the given text. The resulting lists demonstrate the characters that match each sequence character.
Character classes and ranges in regular expressions offer a dynamic way to match specific sets of characters within text. These tools enable you to precisely target and extract data from diverse ranges of characters, enhancing your text processing capabilities.
In this example, character classes and ranges are employed to extract specific sets of characters from the given text. Different patterns like lowercase vowels, digits, letters (both uppercase and lowercase), and hexadecimal digits are matched and extracted using their respective character classes and ranges.
Character class negation within regular expressions introduces a powerful method to match characters that do not fall within a specified set. This allows you to exclude certain characters from your matches, enhancing the precision of your text analysis.
By embracing character class negation, you gain the ability to exclude specific character types from your matches, ensuring your text analysis remains flexible and targeted.
Let’s delve into a practical example that demonstrates how character class negation can be used to exclude specific character sets from matches.
In this example, character class negation is employed to exclude specific character sets from the matches. The resulting lists showcase the characters that do not fall within the specified character classes. This approach allows you to target and manipulate data precisely, excluding certain character types as needed.
Quantifiers in regular expressions provide the means to specify how many times a character or group should occur in your text. This dynamic feature enhances your ability to target and extract data more efficiently, adapting to various text patterns effortlessly.
By embracing quantifiers, you can fine-tune your text matching to capture different patterns with ease, making your text analysis process more versatile and accurate.
Quantifiers in regular expressions enable you to match repetitive occurrences of characters or groups within text. This empowers you to efficiently locate and extract data with varying repetition patterns.
Leveraging quantifiers for matching repetitions allows you to precisely target and extract data that adheres to specific repetition patterns, making your text analysis more robust and adaptable.
The * quantifier within regular expressions allows you to match patterns with zero or more occurrences of the preceding character or group. This dynamic feature empowers you to capture flexible patterns, from non-existent to repetitive occurrences, enhancing your text analysis capabilities.
In this example, we apply the * quantifier to patterns ‘a*’, ‘e*’, and ‘z*’. The resulting matches show sequences with zero or more occurrences of the respective characters. The pattern ‘a*’ matches a single ‘a’, ‘e*’ matches the sequence of ‘e’s, and ‘z*’ matches nothing, demonstrating the flexibility of the * quantifier in capturing various patterns.
The + quantifier in regular expressions empowers you to match patterns with one or more consecutive occurrences of the preceding character or group. This versatile tool enables you to capture patterns that must appear at least once, enhancing your ability to pinpoint meaningful data within text.
In this example, we use the + quantifier with patterns ‘b+’, ‘c+’, and ‘d+’. The resulting matches demonstrate sequences with one or more occurrences of the respective characters. The pattern ‘b+’ captures both single and consecutive ‘b’ characters, while ‘c+’ captures the single ‘c’, and ‘d+’ captures the single ‘d’, showcasing the functionality of the + quantifier in identifying meaningful patterns.
The ? quantifier in regular expressions enables you to match patterns with either zero or one occurrence of the preceding character or group. This versatile tool accommodates optional elements within your text, allowing you to capture variations without strict presence requirements.
In this example, we utilize the ? quantifier with patterns ‘u?’, ‘o?’, and ‘l?’. The resulting matches showcase sequences with zero or one occurrences of the respective characters. The pattern ‘u?’ captures the optional ‘u’, ‘o?’ captures the optional ‘o’, and ‘l?’ captures the optional ‘l’, highlighting the versatility of the ? quantifier in accommodating variations within your text.
The {m, n} quantifier in regular expressions allows you to specify a custom range for the number of occurrences of the preceding character or group. This gives you precise control over matching patterns with a minimum of m occurrences and a maximum of n occurrences, offering adaptability in capturing varied data.
In this example, we apply the {m, n} quantifier to patterns ‘a{2,4}’, ‘b{1,3}’, and ‘c{0,2}’. The resulting matches demonstrate sequences with a custom range of occurrences of the respective characters. The pattern ‘a{2,4}’ captures sequences with 2 to 4 consecutive ‘a’ characters, while ‘b{1,3}’ and ‘c{0,2}’ do not find any matches within the given text. This showcases the precision and flexibility of the {m, n} quantifier in customizing your matches.
Special characters in regular expressions play a crucial role in defining complex patterns and enhancing your text matching capabilities. These characters enable you to pinpoint specific positions, sequences, and structures within text, enabling you to extract meaningful data with precision.
By mastering the usage of these special characters, you gain the ability to construct intricate patterns that efficiently capture and manipulate data according to your specific requirements.
In this example, we demonstrate the application of special characters in regular expressions. We use .* to match any sequence of characters after the “Hello! ” greeting, \d+ to match digits, ^ to match words at the beginning of lines, and $ to match words at the end of lines. These special characters enhance the precision and effectiveness of your text matching and extraction tasks.
Escaping special characters in regular expressions involves adding a backslash (\) before them. This preserves their literal meaning rather than treating them as part of the regular expression syntax. Escaping is essential when you want to match these special characters exactly as they appear in the text.
By escaping special characters, you ensure that they are interpreted as regular characters and not part of the regular expression syntax, allowing for accurate matching within your text data.
In this example, we escape the special characters $, (, ), and \ using the backslash (\) to ensure their literal interpretation in the regular expression pattern. This allows us to accurately match these special characters within the given text.
Anchors in regular expressions provide a way to match patterns that are constrained to specific positions within the text. They help you ensure that a pattern occurs at the beginning (^) or end ($) of a line, allowing you to precisely target data within defined boundaries.
By utilizing anchors, you gain control over where a pattern should appear in your text, enhancing the accuracy of your text matching and extraction tasks.
In this example, we use anchors to match lines that start with ‘Hello’ and end with ‘fine.’. The ^ anchor ensures that the pattern occurs at the beginning of a line, while the $ anchor enforces the pattern to be at the end of a line. Anchors help you navigate and extract data based on their specific positions within the text.
Word boundaries in regular expressions allow you to define precise boundaries for word matching. They enable you to target patterns that appear at the beginning or end of words, ensuring accurate matches without including partial or overlapping words.
\bpattern\b: Matches pattern only when it forms a whole word.
By utilizing word boundaries, you can extract or manipulate specific words within your text without mistakenly including substrings that share partial matches with your target pattern.
Here’s a Python code snippet that illustrates the use of word boundaries (\b) in regular expressions to match patterns as whole words:
In this example, we use word boundaries (\b) to match the whole words ‘cat’, ‘category’, and ‘cute’. The use of word boundaries ensures that only the desired complete words are matched, preventing partial matches from being included. This guarantees precision in word matching within your text.
In this first part of our exploration into regular expressions, we’ve delved into the fundamental concepts that serve as the building blocks for harnessing the immense power of text pattern matching.
As we conclude this first part of our journey, remember that these concepts form the foundation for a deeper understanding of regular expressions. In the upcoming second part of our exploration, we’ll dive even deeper into advanced topics, including quantifiers, character classes, and more. So stay tuned for Part 2, where we’ll continue to unravel the full potential of regular expressions and equip you with the tools to tackle even more complex text pattern. Follow 1stepgrow if you enjoyed reading the blog.
We provide online certification in Data Science and AI, Digital Marketing, Data Analytics with a job guarantee program. For more information, contact us today!
Courses
1stepGrow
Anaconda | Jupyter Notebook | Git & GitHub (Version Control Systems) | Python Programming Language | R Programming Langauage | Linear Algebra & Statistics | ANOVA | Hypothesis Testing | Machine Learning | Data Cleaning | Data Wrangling | Feature Engineering | Exploratory Data Analytics (EDA) | ML Algorithms | Linear Regression | Logistic Regression | Decision Tree | Random Forest | Bagging & Boosting | PCA | SVM | Time Series Analysis | Natural Language Processing (NLP) | NLTK | Deep Learning | Neural Networks | Computer Vision | Reinforcement Learning | ANN | CNN | RNN | LSTM | Facebook Prophet | SQL | MongoDB | Advance Excel for Data Science | BI Tools | Tableau | Power BI | Big Data | Hadoop | Apache Spark | Azure Datalake | Cloud Deployment | AWS | GCP | AGILE & SCRUM | Data Science Capstone Projects | ML Capstone Projects | AI Capstone Projects | Domain Training | Business Analytics
WordPress | Elementor | On-Page SEO | Off-Page SEO | Technical SEO | Content SEO | SEM | PPC | Social Media Marketing | Email Marketing | Inbound Marketing | Web Analytics | Facebook Marketing | Mobile App Marketing | Content Marketing | YouTube Marketing | Google My Business (GMB) | CRM | Affiliate Marketing | Influencer Marketing | WordPress Website Development | AI in Digital Marketing | Portfolio Creation for Digital Marketing profile | Digital Marketing Capstone Projects
Jupyter Notebook | Git & GitHub | Python | Linear Algebra & Statistics | ANOVA | Hypothesis Testing | Machine Learning | Data Cleaning | Data Wrangling | Feature Engineering | Exploratory Data Analytics (EDA) | ML Algorithms | Linear Regression | Logistic Regression | Decision Tree | Random Forest | Bagging & Boosting | PCA | SVM | Time Series Analysis | Natural Language Processing (NLP) | NLTK | SQL | MongoDB | Advance Excel for Data Science | Alteryx | BI Tools | Tableau | Power BI | Big Data | Hadoop | Apache Spark | Azure Datalake | Cloud Deployment | AWS | GCP | AGILE & SCRUM | Data Analytics Capstone Projects
Anjanapura | Arekere | Basavanagudi | Basaveshwara Nagar | Begur | Bellandur | Bommanahalli | Bommasandra | BTM Layout | CV Raman Nagar | Electronic City | Girinagar | Gottigere | Hebbal | Hoodi | HSR Layout | Hulimavu | Indira Nagar | Jalahalli | Jayanagar | J. P. Nagar | Kamakshipalya | Kalyan Nagar | Kammanahalli | Kengeri | Koramangala | Kothnur | Krishnarajapuram | Kumaraswamy Layout | Lingarajapuram | Mahadevapura | Mahalakshmi Layout | Malleshwaram | Marathahalli | Mathikere | Nagarbhavi | Nandini Layout | Nayandahalli | Padmanabhanagar | Peenya | Pete Area | Rajaji Nagar | Rajarajeshwari Nagar | Ramamurthy Nagar | R. T. Nagar | Sadashivanagar | Seshadripuram | Shivajinagar | Ulsoor | Uttarahalli | Varthur | Vasanth Nagar | Vidyaranyapura | Vijayanagar | White Field | Yelahanka | Yeshwanthpur
Mumbai | Pune | Nagpur | Delhi | Gurugram | Chennai | Hyderabad | Coimbatore | Bhubaneswar | Kolkata | Indore | Jaipur and More